KINESSO Ramps Up Scientific Model, Opens 'Experimentation Lab'
by Joe Mandese
Read full article (KINESSO Ramps Up Scientific Model, Opens 'Experimentation Lab')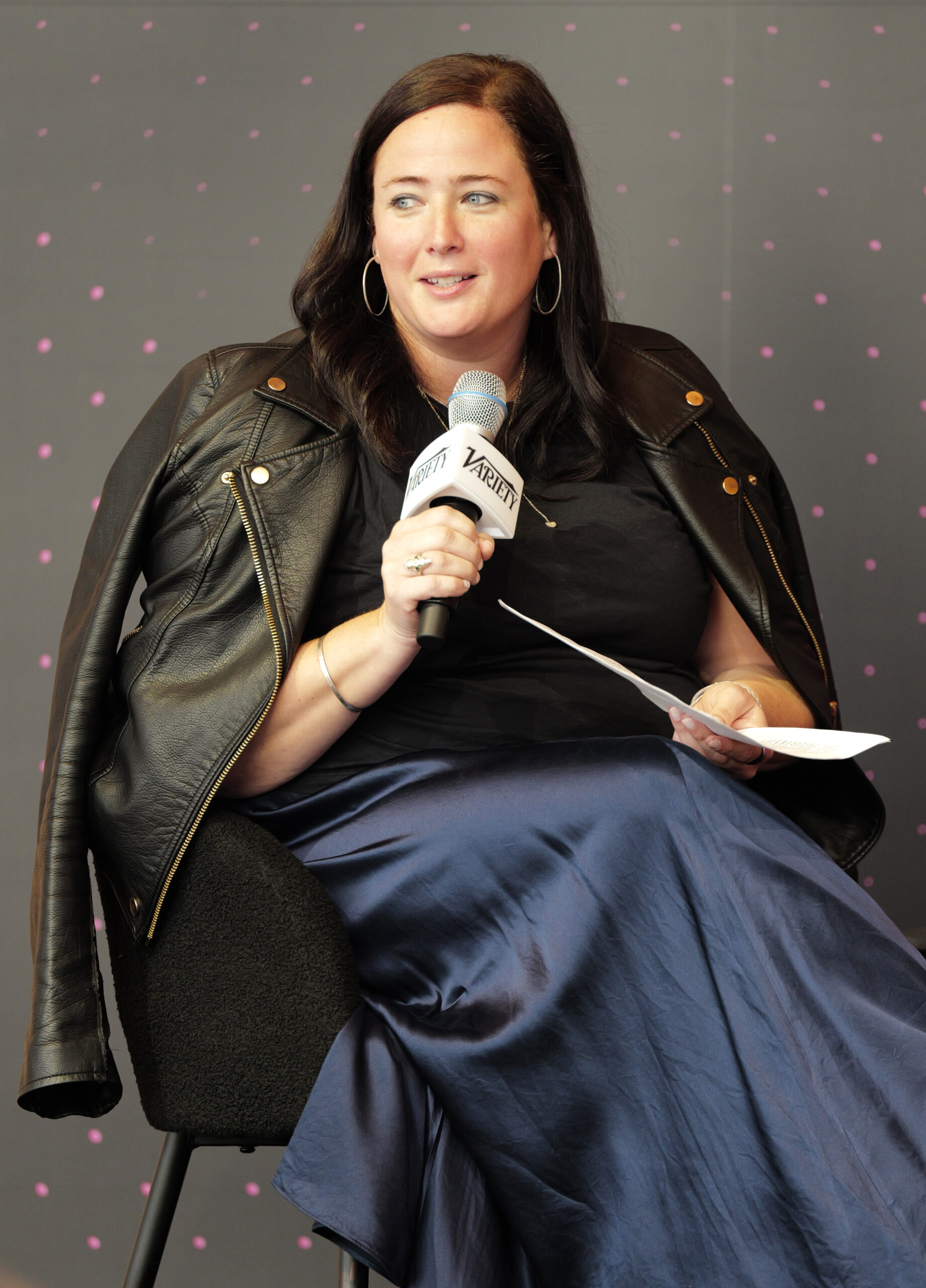
Is it Retail, or is it Commerce
Variety: Content Meets Commerce Summit Presented by KINESSO
Read full article (Is it Retail, or is it Commerce)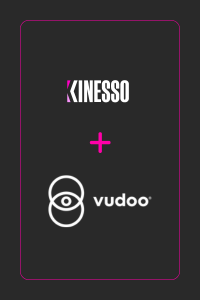
KINESSO Commerce, Partners with Vudoo to Roll Out First-to-Market Global Solution
IPG Mediabrands’ Dedicated Commerce Unit, KINESSO Commerce, Partners with Vudoo to Roll Out First-to-Market Global Solution
Read full article (KINESSO Commerce, Partners with Vudoo to Roll Out First-to-Market Global Solution)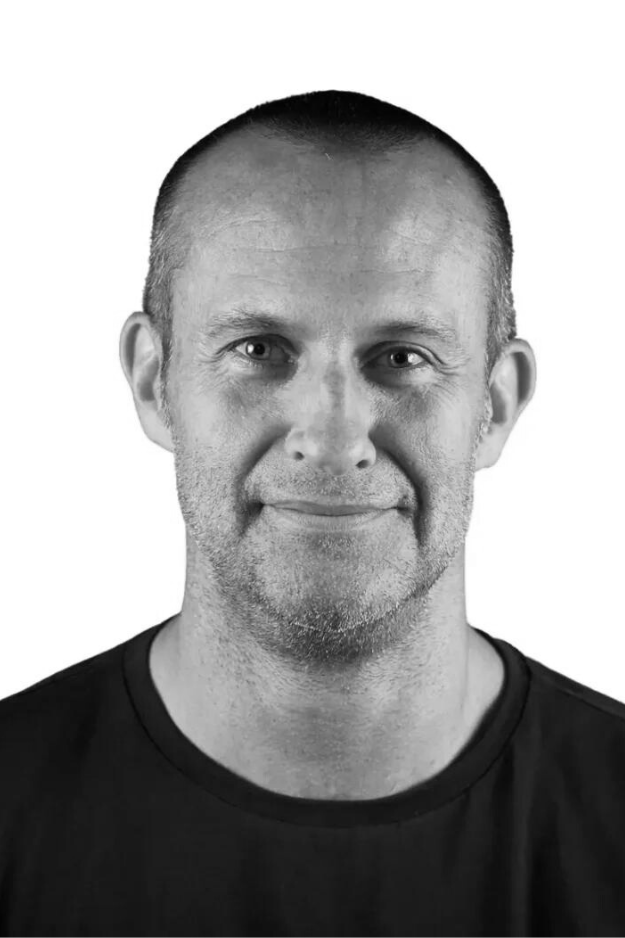
IPG Further Unifies Data, Engineering, and Tech
IPG announces the further unification of its data, engineering, martech and adtech technology resource with Jarrod Martin adding oversight of Acxiom, IPG’s data, identity resolution, and marketing cloud services unit to his remit.
Read full article (IPG Further Unifies Data, Engineering, and Tech)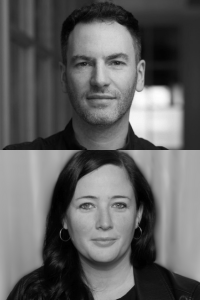
KINESSO Commerce & Global Leadership Moves
The leadership moves come as KINESSO further scales its data & tech expertise across IPG Mediabrands, reinforcing commitment to help brands navigate Commerce, emerging tech & AI
Read full article (KINESSO Commerce & Global Leadership Moves)